Summary: Researchers harnessed the power of artificial intelligence (AI) to measure the fluid flow around the brain’s blood vessels, a major breakthrough with far-reaching implications for treating diseases like Alzheimer’s.
The team developed innovative AI velocimetry measurements to calculate this fluid flow accurately. The newly-developed technique combines 2D measurements with physics-informed neural networks to provide high-resolution insights into the system.
This study opens a new frontier in understanding the mechanisms driving brain fluid flow.
Key Facts:
- The multidisciplinary team that developed the AI velocimetry measurements is a unique combination of mechanical engineers, neuroscientists, and computer scientists, exemplifying the importance of cross-disciplinary collaboration in advancing scientific understanding.
- The researchers were able to generate a three-dimensional model of the fluid flow in the perivascular spaces around the brain. This represents a significant advancement from previous two-dimensional studies, adding a layer of complexity that more accurately reflects the intricacies of the human brain.
- The AI-based technique has potential applications beyond Alzheimer’s research. Understanding fluid flow dynamics around the brain can also assist in developing treatments for other conditions linked to fluid flow alterations, such as small vessel disease, strokes, and traumatic brain injuries.
Source: University of Rochester
A new artificial intelligence-based technique for measuring fluid flow around the brain’s blood vessels could have big implications for developing treatments for diseases such as Alzheimer’s.
The perivascular spaces that surround cerebral blood vessels transport water-like fluids around the brain and help sweep away waste. Alterations in the fluid flow are linked to neurological conditions, including Alzheimer’s, small vessel disease, strokes, and traumatic brain injuries but are difficult to measure in vivo.
A multidisciplinary team of mechanical engineers, neuroscientists, and computer scientists led by University of Rochester Associate Professor Douglas Kelley developed novel AI velocimetry measurements to accurately calculate brain fluid flow.
The results are outlined in a study published by Proceedings of the National Academy of Sciences.
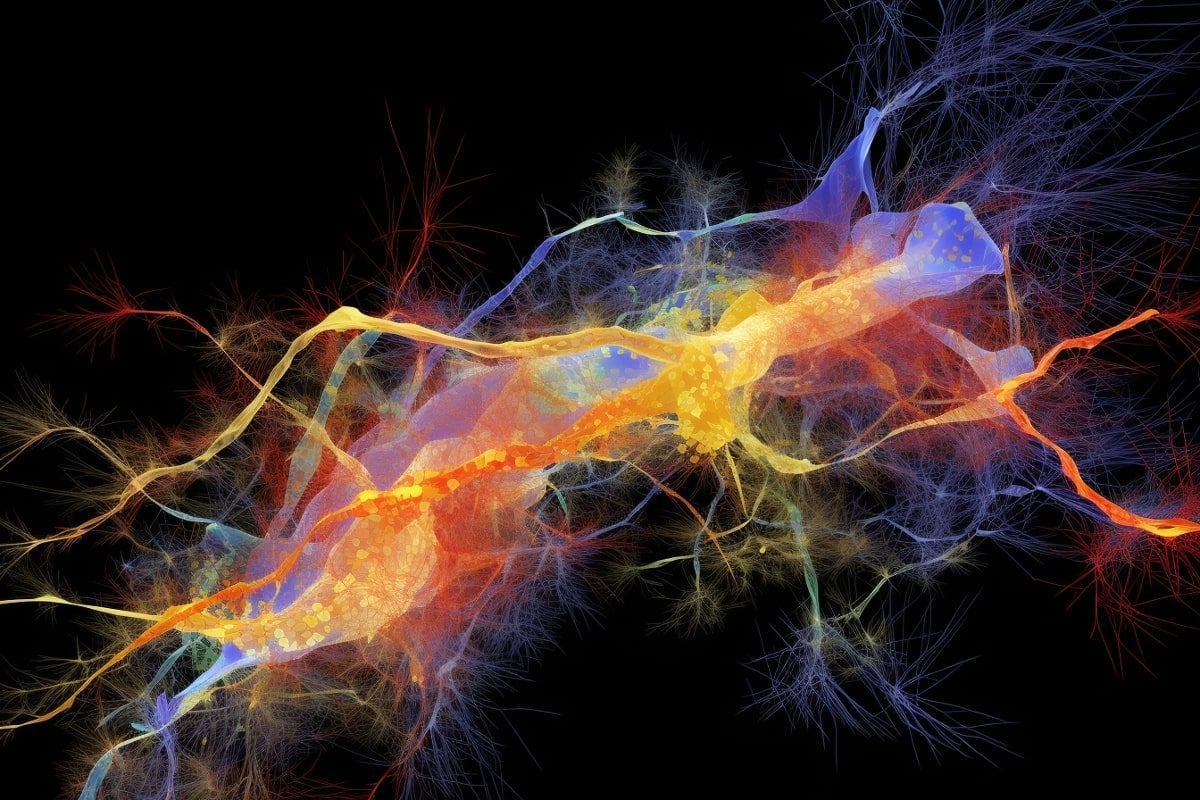
“In this study, we combined some measurements from inside the animal models with a novel AI technique that allowed us to effectively measure things that nobody’s ever been able to measure before,” says Kelley, a faculty member in Rochester’s Department of Mechanical Engineering.
The work builds upon years of experiments led by study co-author Maiken Nedergaard, the codirector of Rochester’s Center for Translational Neuromedicine.
The group has previously been able to conduct two-dimensional studies on the fluid flow in perivascular spaces by injecting tiny particles into the fluid and measuring their position and velocity over time.
But scientists needed more complex measurements to understand the full intricacy of the system—and exploring such a vital, fluid system is a challenge.
To address that challenge, the team collaborated with George Karniadakis from Brown University to leverage artificial intelligence. They integrated the existing 2D data with physics-informed neural networks to create unprecedented high-resolution looks at the system.
“This is a way to reveal pressures, forces, and the three-dimensional flow rate with much more accuracy than we can otherwise do,” says Kelley.
“The pressure is important because nobody knows for sure quite what pumping mechanism drives all these flows around the brain yet. This is a new field.”
About this AI and Alzheimer’s disease research news
Author: Douglas Kelley
Source: University of Rochester
Contact: Douglas Kelley – University of Rochester
Image: The image is credited to Neuroscience News
Original Research: Closed access.
“Artificial intelligence velocimetry reveals in vivo flow rates, pressure gradients, and shear stresses in murine perivascular flows” by Douglas Kelley et al. PNAS
Abstract
Artificial intelligence velocimetry reveals in vivo flow rates, pressure gradients, and shear stresses in murine perivascular flows
Quantifying the flow of cerebrospinal fluid (CSF) is crucial for understanding brain waste clearance and nutrient delivery, as well as edema in pathological conditions such as stroke.
However, existing in vivo techniques are limited to sparse velocity measurements in pial perivascular spaces (PVSs) or low-resolution measurements from brain-wide imaging. Additionally, volume flow rate, pressure, and shear stress variation in PVSs are essentially impossible to measure in vivo.
Here, we show that artificial intelligence velocimetry (AIV) can integrate sparse velocity measurements with physics-informed neural networks to quantify CSF flow in PVSs. With AIV, we infer three-dimensional (3D), high-resolution velocity, pressure, and shear stress.
Validation comes from training with 70% of PTV measurements and demonstrating close agreement with the remaining 30%. A sensitivity analysis on the AIV inputs shows that the uncertainty in AIV inferred quantities due to uncertainties in the PVS boundary locations inherent to in vivo imaging is less than 30%, and the uncertainty from the neural net initialization is less than 1%.
In PVSs of N = 4 wild-type mice we find mean flow speed 16.33 ± 11.09 µm/s, volume flow rate 2.22 ± 1.983 × 103 µm3/s, axial pressure gradient ( − 2.75 ± 2.01)×10−4 Pa/µm (−2.07 ± 1.51 mmHg/m), and wall shear stress (3.00 ± 1.45)×10−3 Pa (all mean ± SE).
Pressure gradients, flow rates, and resistances agree with prior predictions. AIV infers in vivo PVS flows in remarkable detail, which will improve fluid dynamic models and potentially clarify how CSF flow changes with aging, Alzheimer’s disease, and small vessel disease.
Resource: Neroscience News